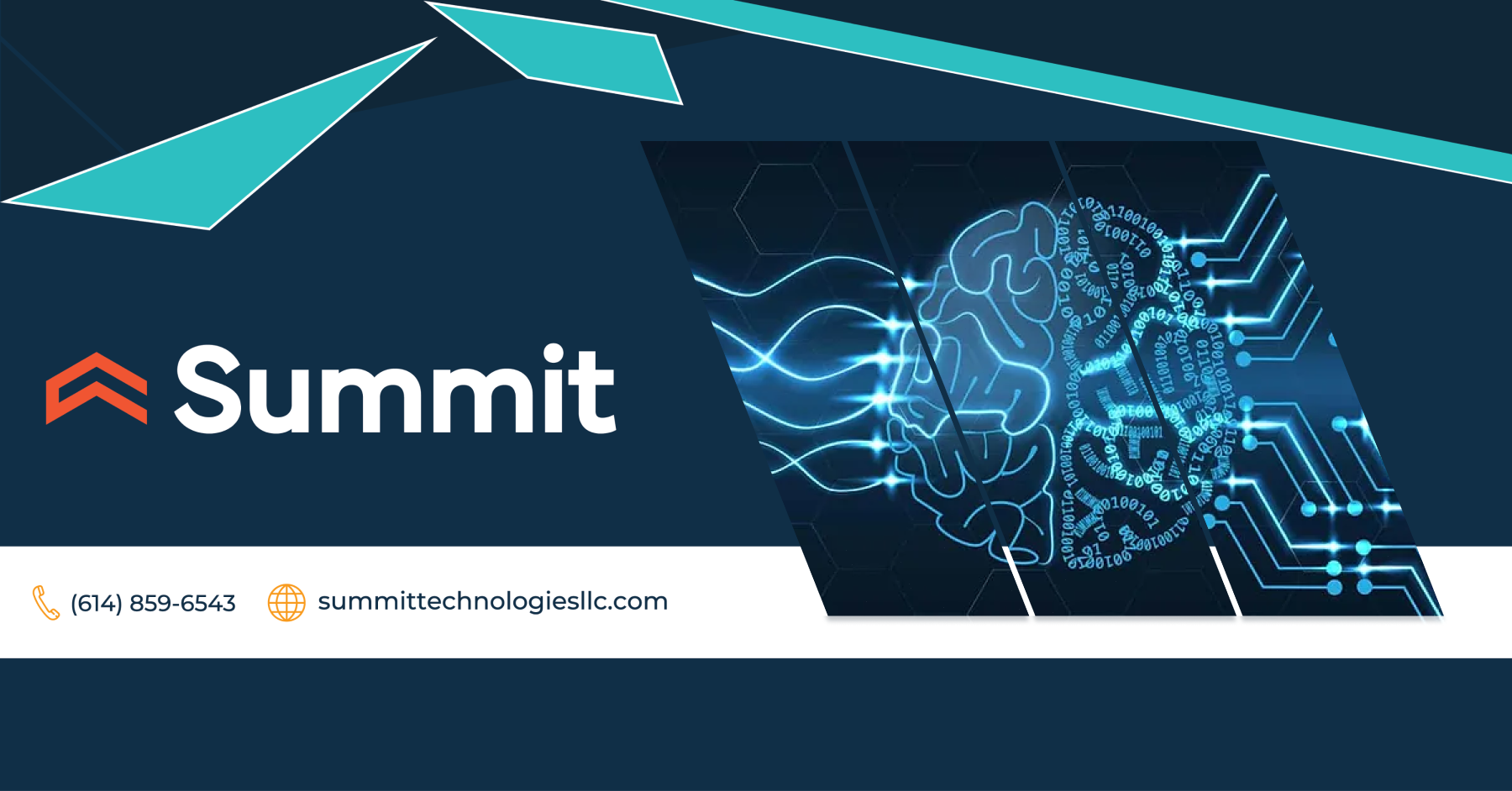
Artificial Intelligence may seem a bit mysterious, maybe a little magical, when you first hear about what it can do. The truth is that the AI that powers business applications really boils down to a few small parts which, when combined in innovative ways, delivers real value for businesses. One way to think about artificial intelligence is as ingredients that come together to create a perfect recipe for your business. Combine the ingredients one way and you get one dish. Combine the ingredients in a different way and you get a different dish. These simple ingredients can lead to an impressive variety of solutions for your business needs.
Let’s take a look at the four main ingredients that are part of any good AI platform: yes-and-no predictions, numeric predictions, classifications, and recommendations.
Yes-and-No Predictions
The first ingredient is yes-and-no predictions. Yes-and-no predictions allow you to answer questions like, “Is this a good lead for my business?” or “Will this prospect open my email?” AI helps you answer these questions by scanning historical data you’ve stored in your system.
Yes-and-no predictions generally come in the form of a probability (for example, “Mary Smith has a 67% chance of opening this type of email). But sometimes probabilities are converted into scores. Scores are just a different representation of the likelihood of “yes”; they can be represented as numbers on a numeric scale (for example, 0 to 100) or even as the number of stars on a five-star rating survey. Keep in mind that these scores are just showing the same probability in a different way.
Numeric Predictions
Next, are numeric predictions. Numeric predictions often power predictive forecasting solutions (for example, “How much revenue will this new customer bring in?”), but they are also used in other contexts like customer service (for example, “How many days will it take us to resolve this customer’s issue?”). Numeric predictions also use your historical data to arrive at these numbers.
Classifications
Next, we have classifications. Classifications frequently use “deep learning” capabilities to operate on unstructured data like free text or images. The idea behind classification is to extract useful information from unstructured data and answer questions like, “How many soda cans are in this picture?” It can even take a statement like, “I’d like to buy another pair of the same shoes I bought last time,” and use that to kick off a workflow that can look up the last shoe order and place the same pair of shoes in their online shopping cart.
Classification using deep learning is very robust, even when the unstructured data is arriving in different forms. Take the previous example of shoes. You could just say, “I want another pair of those shoes” or “Give me another one of those.” No matter how the request is phrased, the underlying deep learning engine built into an AI platform can generally understand them all, in much the same way that your brain can.
Another type of classification—which may or may not use deep learning—is called clustering. This type of AI ingredient gathers insights from your data that you may not otherwise have noticed. For example, if you are a clothing vendor, AI might learn that both rural older men and urban twentysomethings like to buy a certain type of sweater. Where your intuition might tell you that these are two totally different groups, the data shows they behave similarly with respect to the products they buy, and you may want to market to those two groups in a similar way.
Recommendations
Last, are recommendations. Recommendations are key when you have a large set of items that you’d like to recommend to users. Many ecommerce websites apply recommendation strategies to products; they can detect that people who bought a specific pair of shoes also often order a certain pair of socks. When a user puts those shoes in their cart, AI automatically recommends the same socks.
Recommendations are not just for products. Marketers use the same technique to recommend content like whitepapers to business users. Employers might use recommendations with their HR recruiting system to recommend job postings to job candidates.
Workflow and Rules
Workflow and rules aren’t technically part of AI, but they’re an essential part of how AI is used. Take the following example. Let’s say that AI predicts a given customer has a 25% likelihood of not renewing their contract. Just knowing this is not enough—you need to do something about it. That’s where workflow and rules come into play. In this example, your workflow might mean kicking off a retention campaign when the AI predicts that a customer is unlikely to renew.
With these four fundamental AI ingredients, you can produce custom AI applications that meet a variety of business needs.