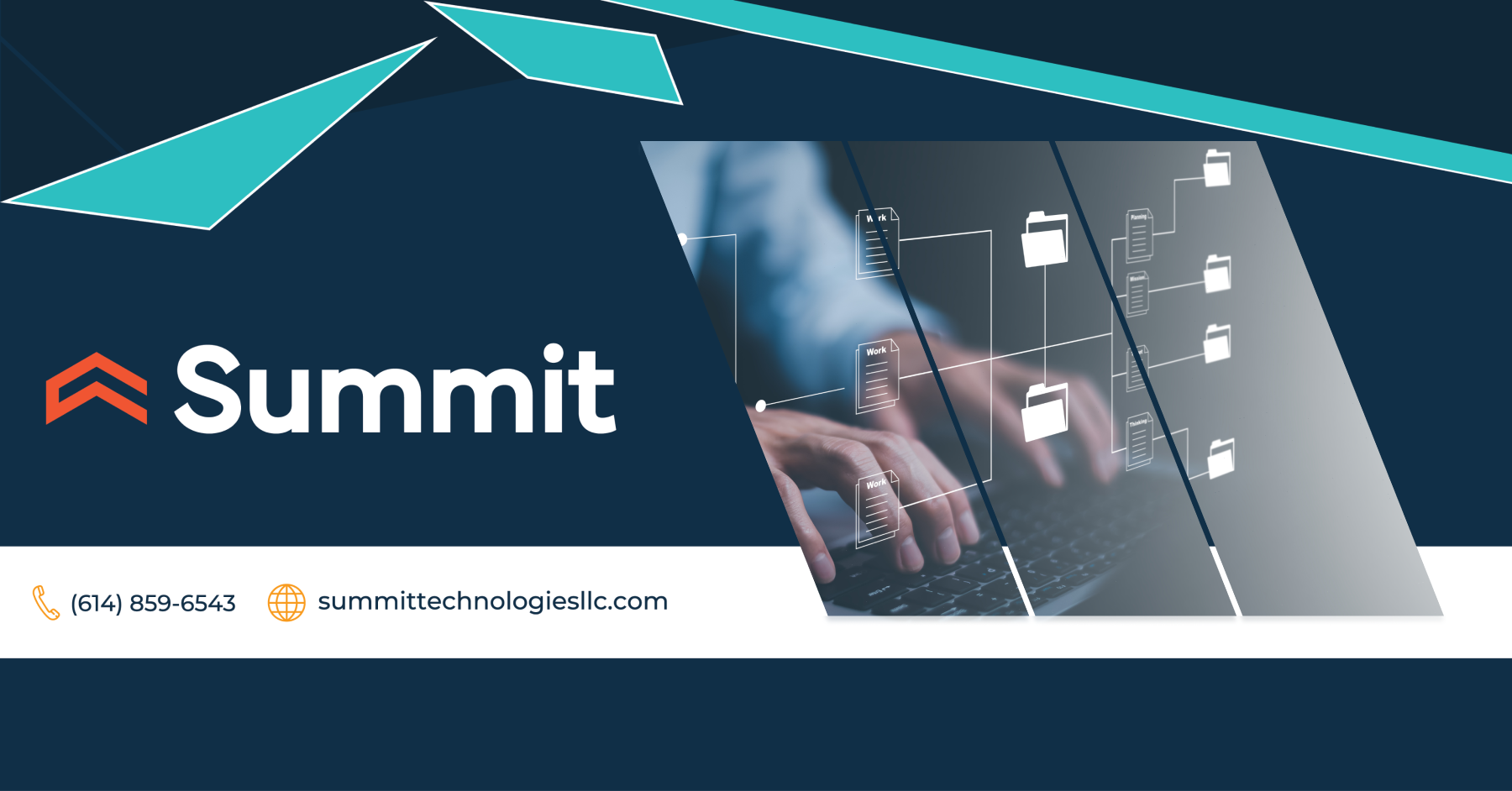
You know what data is: facts and figures and info. Your data is the who, what, where, and when of your business. And you know what analytics is: analysis involves looking at facts and coming to conclusions. Analytics gives you the why of your business.
If you have been collecting data for a while you might be wondering if it is now big data. Big data is the nearly uncountable things in that vast sea of info going around on computers and the Internet. Big data even includes information not directly related to your business but relevant to your business. You might think of it as the why not of your business. Big doesn’t even begin to describe the petabytes of information our society is creating! But when you’re looking for interesting conclusions and meaningful analyses, digging in to big data is where you’ll find what you need.
Big data is “big” for many reasons. One is its sheer volume. The amount of information is increasing in leaps and bounds, far beyond what we thought possible just a few years ago. Getting your arms around that much data can be a challenge.
Another reason big data is big is because it is changing so rapidly. Imagine that a bank is collecting data on its customer transactions. That’s a lot of data, but the information isn’t standing still. More data is being added all the time, at increasing velocity.
Lastly, big data is complicated because it gets collected in a variety of different formats. Data like a banking transaction is relatively easy to analyze, but what if it’s combined with the emails the bank receives, the likes the daily bank blog gets, and statistics about the companies the bank’s customers do business with? Trying to analyze all of this data together is like trying to figure out the individual ingredients of a meal you just enjoyed.
For all these reasons, big data is more challenging to analyze. But, the rewards are also much bigger. Analyzing large sets of information can reveal previously invisible insights that are hidden in the chaos of big data.
In the past, data analytics was limited, literally, by the size of the data. A hard drive, thumb drive, or CD could only hold a limited amount of information. Even if that number increased, there was always more data available than the device could hold.
You had to compromise your analysis to fit the storage limitations. Either you used a subset of your data, or only used the most recent data. No matter what, you had to choose. And trying to figure out why last quarter’s sales went down by hacking at a spreadsheet with year-old data would be like trying to drive while only looking at the rear-view mirror.
With cloud computing, you no longer have to choose between having room to store more data, and getting insights. Because you pay for what you use, rather than having to maintain your hardware, and you can pay as you go, it’s easy to add more capacity when you work with data in the cloud rather than your own IT room. And more data from more sources means better analyses.
It’s rare to only have one person analyzing data, especially big data. So how do you know whose analysis is the most recent? When everybody is working in their own spreadsheets, it can be nearly impossible to tell. But looking at data stored in a cloud means you can keep everyone’s work up to date and all your colleagues in sync.
It’s fair to ask: between the sheer volume of the data and complexity of analyzing it, do you need a PhD in math to do data analytics? Luckily, the answer is no! With the right technology, you can look at the data yourself and create your own analysis-without an advanced degree.
What are some typical tools for data analytics? Software in the cloud is one super useful tool for analyzing big data. Of course, different software packages have varying strengths and pitfalls. With Salesforce Einstein, you can:
Never run out of space:
- Einstein scales for your data.
- Find an option to meet your needs, since there are a variety of Einstein products
- Automatically discover relevant patterns
- See patterns in your data visually with Einstein and Tableau
You’ve probably heard the saying that you need to measure what’s important. The same thing is true about data analytics. What questions do you want to answer with your analysis? What are the whys and why nots you need to know?
Two typical kinds of questions lead to descriptive and prescriptive analytics. What’s the difference? One analyzes the past, and the other looks to the future.
Nonprofit and education leaders are often driven by the numbers used for descriptive analytics. How many donations did our latest giving campaign get? Did our postcards to people who completed the SAT drive more applications to our university? Are numbers of recurring donors going up? Descriptive analytics answers these questions. Typically, they involve a period of time-a month, a semester, or a year.
Prescriptive analytics, on the other hand, asks about what should be done in the future. Based on past data, this kind of analysis suggests a course of action. For example, imagine that you have data on student graduation rates, along with other factors like if they paid their tuition on time, if they logged in to the student community hub, if they met with their advisor at least once per semester, and so on. Using this data, you can see what factors influenced graduation rates, and then take the next best action to improve the situation.
If you then learn that if students are logging in to the student community and visiting their advisors more often, you can plan recommendations to push SMS text messages or send reminders to students to take actions to help them. Your analysis led to a next best action, or recommendation, for what to do in the future.
There are as many types of answers as there are questions. And data analytics can get you there!